Disclaimer
This paper is a chapter-preview of the book “Big Data for Culture and Tourism” that will be published, by the Rossi Smith Academic Publishing in the second half of 2018.
Abstract
The Big-Data revolution has been immediately accepted in several industries, in particular the military and defense industries, by various economic and social actors, usually linked to multinational corporations, as a fresh source of innovation. In the cultural sector, these new technologies acceptance has been less common met some oppositions.
These oppositions have been mainly due to a general lack of competences on the new technologies and on advanced data analysis by most of the actors in this sector.
Lacking the needed competences, these actors have often been unable to grasp the opportunities that Big-Data could have opened to them.
In this paper, we propose to highlight the main results and main positive effects coming out from the first stages of Big-Data utilization in the Cultural Heritage sector using a fractal model.
Introduction
Big-Data is surely one of the modern days’ buzz-words based on the idea of a decision making revolution linked to the analysis of very large data sets (Boyd & Crawford, 2011). The modern data set are “big” in all the three dimensions identified by Laney (2001) as the 3 V’s: Volume (the quantity of data a company has access to), Velocity (the speed of data gathering processes), and Variety (the multiple types of data stored).
Moreover, modern day Big-Data are mostly based upon the actors’ real behaviors and, as such, they are more trustworthy even if nested in complex structures (Ward & Barker, 2013).
Meanwhile, it is becoming manifest that Big-Data analytics are not a fad, but they are more useful the more the new technologies (in terms of data storage, bandwidth, and accessibility) drive human beings to rely on the Internet as a communication media, as a way to find new information, and as a way to easily store and share the memories of each single experience they make (Pine & Gilmore, 1999; Aiden & Michel, 2014). The relevance of Big-Data analytics is becoming increasingly apparent in some fields as digital marketing, and business intelligence, where enterprises can leverage the new ICTs to engage their audience more effectively, to foresee the new opportunities, and to create a long-term relationship with consumers (Sashi, 2012).
Big-Data analysis has become common in several industries as the access to new, and richer set of data can help managers to embrace a data-driven decision making process that can increase their choices effectiveness (Lazer et al., 2009; McAfee & Brynjolfsson, 2012; De Mauro et al., 2015).
At the same time, other industries appear to be mostly impervious to the adoption of Big-Data analysis; the Cultural Heritage sector is surely among them.
The general lack of adoption of Big-Data driven decision making processes sounds even stranger when it is considered that Cultural Heritage sector is tightly linked with the Tourism Destination and its management. Cultural Heritage is usually considered among the “core resources and attractors” of a destination (Crouch & Ritchie, 1999, p. 146) – i.e. they are among the reasons tourists travel to a tourism destination. According to Crouch and Ritchie (1999) a destination is able to effectively attract tourists as these resources are coupled with other resources, called support resources, in order to increase their appeal to tourists.
Destination Management Organizations have adopted Big-Data analytics to understand the reasons behind tourist flows in specific locations as parks (Wood et al., 2013) or cities (Buhalis & Amaranggana, 2013) leveraging the mass of data that tourists themselves autonomously provide trough social media.
In this paper, we propose a framework to highlight how Big-Data can help the Cultural Heritage sector to become more effective, analyzing the main results and positive effects coming out of the first stages of Big-Data utilization in the Cultural Heritage sector.
Big-Data to create value for the area and for its heritage
Competition in tourism industry is getting fiercer as places become viable as Tourism Destination (Mariani et al., 2014). It appears clear that, in this competitive landscape, the players feel the real value behind getting a better understanding of actual and future tourists’ needs, to reach a better competitive position (Wang et al., 2013). In the process to gather tourist needs data, the operators can leverage the many traces that tourists leave on the Internet from the first moment of planning the travel to those they create while they are experiencing the tourism destination (Li & Buhalis, 2006; Xiang & Gretzel, 2010).
Big-Data offers tourism operators the opportunity to get access to a deeper knowledge of tourists, actual and potential ones, and it helps them in engaging all the players, even those external to the destination itself, in creating services with a better value proposition for the tourists (Pike, 2005; Wang et al., 2013).
Analyzing these large data sets can help the tourism operators in creating preferred services that are better aligned to the tourist perspective of their travel as a whole (Franch, 2002; Rossi, 2008). Moreover, having access to these data can help the tourism operators in defining strategically the place’s core and support resources (Crouch & Ritchie, 1999) limiting the investments in those services that are not able to increase the value of the destination for its visitors.
Adopting Big-Data analytics, place managers can profile tourists and highlight the various latent opportunities normally not exploited in the local area to create new services attractive to different classes of tourists. In this way, they can increase the effectiveness of the local area in the international market (Palmer & McCole, 2000; Getz, 2008; Buhalis & Law, 2008).
On the other hand, operators can use Big-Data to profile the tourists, to predict, and to understand, their evolution over time. This knowledge can later be used to help in focusing the place development efforts on the more relevant core resources of the area and in creating the needed support resources to make the most out of them (Seddighi & Theocharous, 2002).
At the same time, we have to take into consideration that the area can be considered as a complex system of different variables at different levels so the effect of Big-Data analytics on a place has to be analyzed at various levels (Allen & Sanglier 1979; Warnaby et al. 1998).
At the macro-level, i.e. looking at the relationship between the various destinations, Big-Data analytics can be used to create value from the sharing of the datasets created in the various destinations as a way to define a coopetitive approach (Brandenburger & Nalebuff, 1996). Where each destination, as a whole, can get access to the data the tourists have created in their experiences in the other destinations as a source of information to create new, and better, services that will be appealing to tourists that have chosen to visit different destinations in the past. The internet-based nature of most of the data on the tourist behavior (i.e. post and messages on social networks and similar services) reduce the need for a complex coordination at the macro-level. The value of these common data can be increased if they can be combined with those gathered using the device in the so-called Internet of Things – i.e. sensors and devices connected on line – in order to monitor tourists behaviour during their visit and to provide them with personalized services (Solima et al. 2016).
Meanwhile, in order to get the most out of these processes, the local actors must be able to co-ordinate with other actors outside of the local area (for example an Hotel in the local area as to be able to coordinate with a Tour Operator at distance). In order to make their services accessible to a set of tourists as broad as possible, and to win their loyalty to the place (Fyall et al. 2003).
A shared access to Big-Data can be useful at the meso-level, i.e. all those actors operating in the place, as it makes them reach higher level of coordination to create bundles of services, tourism packages, that, being more precisely targeted on the need of the tourist, can lead to better experiences and, therefore, they can more easily satisfy the tourist (Neal & Gursoy, 2008; Bernè et al. 2015).
The ability to coordinate between the various actors in a given tourism destination can be one of the main factors in obtaining the most out of strategic alliances in tourism (Della Corte & Aria, 2014) and of the ability of tourism destination to compete in the global market (Della Corte & Aria, 2016).
The potential of creating new value at the level of the whole tourism destination is greater the more the various players can get access to a diversified set of resources to use as the building blocks of their packages (Andreu et al., 2010).
In this perspective, the operators in the Cultural Heritage sector can become part of a broader coordination attempt as the other players in the local area can create stable relationships with them in order to reach a different set of tourists.
The resources the players in the Cultural Heritage sector can leverage in their services can give the local area several strategic advantages as the cultural resources are, usually, tightly linked to the area, its history and its culture, while, on the other hand, their exploitation is not limited to a specific part of the year (i.e. summer or winter) and/or they can be organized in events (i.e. festivals) that can be used to offer tourism services in all months of the year increasing the efficiency of the investments of the other actors in the area.
Finally, even each single player, i.e. the micro-level of the systemic perspective, in the tourism industry can benefit from a general access to the new knowledge generated by these analyses as being more aware of the needs of the tourists and of their evolution. Tourism operators can decide to change their services in order to become more appealing to some specific niche of tourists using differentiation strategies to make their specific environment a less competitive one.
At the micro-level, the access to Big-Data helps in creating a smart approach to tourism experiences that leverage the idea of creating personalized services (Buhalis & Amaranggana, 2015).
Big-Data and Museum Institutions
According to Nuccio and Bertacchini (2016), Big-Data analytics, in combination with ICT technologies, open the way to a new generation of cultural services that have the potential to enhance greatly visitors’ participation in cultural activities from the museum organizations. The access to new market niches is only one of the advantages these organization can obtain from Big-Data, another advantage is to get access to new ways to track and analyse how visitors perceive, and experience, these organization’s services directly from their behavior during the visit itself. According to Chun (2016), museum organization can create new services that can use ad-hoc created devices to gather even some bio-feedback from their visitors as the stress levels or the emotions they are experiencing during the visit.
The great potential of these new technologies for museum organizations has been acknowledged by the UK Arts and Humanities Research Council that, in 2014, started several projects to leverage Big-Data analytics in the arts. Among them there’s “The Unusual Suspects” (funded with a little less than £ 185.000) that has seen the cooperation between nine arts and cultural organisations in Newcastle and Gateshead to pool their audience data in order to profile them and identify their main communities as a way to develop the relevant insights needed to choose the most effective ways to engage them and to design innovative services to re-engage the infrequent attendees and to help their activities of audience development as a way to multiply their impact on the local area. Another interesting project the UK AHRC has funded is the “ArtsAPI” (a little short of £ 300.000); this research project aims to create a web-based service to aggregate, analyse and present data from several cultural organization to help them understanding the real value they create, and to show their real impact on local area; moreover, these new knowledge will help them to redefine their business models and value proposition, if necessary.
The aggregation of the data generated by several cultural organization is needed since, according to Manovich (2012), they are still not able to generate on their own Big-Data sets.
Meanwhile, some other museums are trying to leverage the combination of digital technologies and Big-Data as a way to create richer datasets. An example is the Metropolitan Museum of Art in New York that is using digital beacons to track visitors’ behaviour inside its exhibitions. By using these information, the museum has been able to get several advantages: on one hand, it has targeted the merchandise more effectively; on the other hand it has been able to provide customers with more focused information about the exhibited pieces of arts; a third, less obvious, effect has been the opportunity to get a better understanding of the way people use the museum, of their tastes and needs.
Another automatic data gathering experience in a museum has been designed in the Art Institute of Chicago. This museum has installed a network of 300 beacons to collect user data to get heat maps, travel paths and dwell times of the visits. These data have been used to redefine the museum’s exhibitions in order to make them more valuable to the visitor (the AIC has been voted as the No.1 museum in the world for TripAdvisor Travelers in the 2014 and the No.2 in the 2016).
These experiences show that museums can get several advantages from the adoption of Big-Data analytics. Their main effects can derive not only from the creation of more valuable services for the existing visitors; they can be used to create new services that can be attractive to the potential visitors as well. However, these advantages can even go beyond the museum organization itself helping to link it with the other actors in the local area as a way to increase its perceived value.
These effects are stronger when the Big-Data pair with new technologies (Solima, 2016) as a way to automate the data gathering processes, leveraging the personal devices and the visitor behavior in the social media. Not only as they help the museum management to use in their strategic decision making a more in-depth knowledge of the tourist (Solima et al. 2015) while they are helping to increase the visitors’ engagement in the museum activities. The Louvre is an example of using specific sensors in order to gather data on the visitors, and to create new services. The French Museum has installed multiple Bluetooth sensors, compatible with the iBeacon protocol, to monitor the movement of visitors in the exhibitions, provide them with specific needed information in real-time, gather data on their preferences, and design new services (Yoshimura et al. 2014).
A Fractal model to highlight the opportunity of Big-Data analytics in Cultural Heritage
The existing academic literature, and the first experimentations in the Cultural Heritage sector, show that Big-Data analytics can help creating value for tourists and, indirectly, for the local area as well.
The data gathering processes behind Big-Data at least in part designed to engage the tourist. On one side, they leverage the existing new personal devices (i.e. smartphones, and smart watches) to collect automatically the behavioral data of each user, on the other side they leverage the whole mass of user-generated content published in the Internet by the tourists. The first source help in increasing the trust in the data as they leverage on the actual behavior of the user; the second set of sources help to get a more comprehensive picture of the users and, analyzing the various ties they have in social media, these data can be used to identify the community the tourist are part of.
This whole mass of data, coming out of the combination of the various sources can be used to profile the tourists visiting a given destination, to identify their main segments, and to foresee these segment’s potential evolutions.
At the same time, as these data are already on the Internet they can be easily shared as a way to increase their value combining them with those from other organizations.
These positive effects can be used by various actors in the different levels inside the destination to create a continuous process of value enhancement that can be understood using a similar approach.
The literature review has shown that at each different level of the system describing the local area there are similar forces at play that shows how embracing the Big-Data can have similar effect even if at a different scale. In order to highlight the similarities among the various levels of the system we have defined our framework proposals as Fractal. A fractal is a geometric structure where each part as the same characteristics of the whole; they have been already used to model the evolution of complex and chaotic phenomena in physics (i.e. crystal growth), in mathematics (see the field of ERGMs in network theory), organization (Service-oriented communities). A common example of Fractal structure is the Koch Snowflake, shown in the following exhibit 1, where starting from a triangle at each iteration each side of the perimeter get divided in three parts and the middle part get substituted by the other two sides of the equilateral triangle built on it.
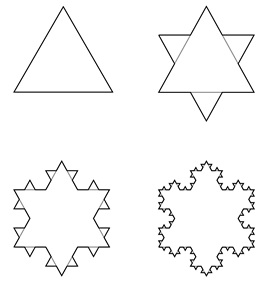
We have labelled our model as a Fractal one, since in each of its levels the overall value proposition made more valuable using two main paths. The first one is to identify a more effective set of support-resources to exploit the part of the system (actor or resource) more effectively; the second one is to link various parts of the level (actors and/or resources) that the tourists consider relevant to create new, different services.
In our framework [1] we have identified four main levels: the cultural resources, the organizations, the various industries, and the destinations.
The first level of this framework is the one of the cultural resources (i.e. the specific pieces of art) inside a specific cultural organization.
The value of these resources for the tourist can be increased finding new, different support resources. An example of this potential evolution are the new, real time, services helping visitors to dynamically get information on a specific piece of art when the visitor is near it; in this way, these new services can help in making the museum accessible to a greater number of visitors thus enhancing its value for the local area.
A further way to increase the value of cultural resources that the managers of a museum have is to coordinate the various core resources to link them in new, different, ways that are able to create more value for a relevant segment of visitors.
Even at the second level, the level composed by all the cultural organizations in the local area, there are two main paths to increase the value of heritage using the knowledge generated using Big-Data.
The first opportunity cultural organization have, is to use the knowledge they have access analyzing the Big-Data to re-design their exhibitions to become more attractive to some new audiences; for example, museum could leverage this knowledge using a smartphone app to advise visitors on specific pieces of art that, according to their behavior, can be of their interest.
A second opportunity to create value at this level is to coordinate the activities designed by the various cultural organizations in the local area as a way to create new diffused services that are able to attract more tourists in the area as a whole.
The various industries in the local area are the actors of the third level of this framework. Big-Data analytics can help the Destination Management to identify the local area more relevant attractors, not only the cultural ones, to use them as the focal element in designing new services and to coordinate the efforts of the various local area actors to create new relationships to make a better value proposition of the area as a whole.
This process does not need to be directed from above; for example, a museum organization can create a stable relationship with those local crafts their visitors value the most in order to sell their products in the bookshop so it can differentiate it from those located in other cultural organizations in the local area. The food that visitors can buy in the buvette, is another example linked to the opportunity to leverage the local area traditions, and culture.
Creating these relationships can help in gathering more data on the tourist habits as different actors from different industries will be able to collect different aspects of the tourists behavior that could be pooled in order to get a well-rounded image of their customers.
This more precise definition of the tourists and of their communities can be used by the Destination management organizations as a driver in their decision making processes on the value propositions of the destination in the global market. Leveraging on data about tourists perception of the destination and on the evolution of their needs over time, these operators will be able to create services to answer to new demand bubbles (Cormiani, 2002).
The last level of this model is the one of the various tourism Destinations. At this level, the actors can increase the value of their services for tourists entering stable relationships with other areas using a co-opetition approach (Brandenburger & Nalebuff, 1996).
A coordination between the various Destination can help in creating a common platform for the data gathering furtherly increasing dataset value.
Obstacles to Big-Data Adoption in Cultural Heritage Organizations
The proposed model highlights that embracing the Big-Data can help Cultural Heritage organizations to create in many different ways a better value proposition. Moreover, the more these organizations are able to get access to relevant resources in the various levels of the model, adding them to their endowments or creating stable relationships with the actors owning them, the more their ability to attract new, potential visitors, grows and the more relevant their impact on the tourism destination attractiveness is.
Meanwhile, we have to acknowledge that in order to adopt entirely the Big-Data paradigm these organizations have to overcome five, meaningful, obstacles.
The first limitation to Big-Data adoption is linked to the need to engage most of their visitors in the data gathering processes. These processes, even the automatic ones, asks the visitors to be an active part; for example, they could be asked to install one or more applications on their smartphones to get access to the services. This links the value of these services to the ability of the cultural organizations to increase digital accessibility of their services.
A second set of limitations are linked to the financial resources needed to install the sensors used to monitor the interactions between the visitor and each piece of art in the exhibitions. Moreover, as the Big-Data effectiveness increases the more the various partners are able to monitor the visitors throughout all their travel, an effective data gathering process needs that several local area operators decide to invest in the installation of a network of sensors.
Then there are the limitations linked to the IT competences that are essential to make cultural organization effective in adopting Big-Data. In order to create an effective data gathering system, these organizations have to be able to align the different computer systems that all the various partners use in a coherent set of services. Bypassing this limitation will asks the cultural organizations to deal with ICT professional able to provide them with the needed competences.
The fourth limitation related to the requirement of developing the required competences to analyse the data gathered and make sense out of them, to profile visitors and to identify the various communities they are part of.
Finally, the theoretical model highlights the need for shared processes among the various local area actors in order to create a biggest possible dataset to leverage in the local area players’ decision making processes. This coordination requires the presence of a local key player able to persuade actors embedded in different levels to cooperate in increasing the overall value of the destination even when they won’t get the related advantages in the short run.
Future opportunities from Big-Data Analysis in Cultural Heritage
This paper has shown that even in their current state the adoption of Big-Data analytics in the Cultural Heritage sector could be able to help the various players in a given local area to cooperate in order to market the destination more effectively and making it develop in a more efficient way.
Meanwhile, we have highlighted that the value of this approach can be reduced by a limited diffusion of the needed technologies among local area operators or amidst tourists.
The modern day evolution of computing appears to help in overcoming both of these factors as people are increasingly active on social media and they frequently use the Internet to share their experiences with their friends.
The increasing adoption of Internet-based services can help to mitigate negative effects of the first and second type of limitations we have highlighted leveraging the diffusion of personal computing devices (i.e. smartphones and smartwatches).
A further relevant factor to consider is the evolution of computing, in particular in the field of Artificial Intelligence. It will help the cultural institutions to analyze in the future these data without having all the advanced data analysis competences necessary today. Considering this evolution in the light of the creation of shared, standard, interfaces, already happening in UK with the development of the ArtsAPI, will help reducing the negative effects related to this limitation (i.e. the lack of IT competences in cultural organizations).
Footnotes
[1] This framework adopts the perspective of the different ways to enhance the value of Cultural Heritage in a destination; a similar approach could be used to describe the relationship starting from the other resources of the destination.
References
Aiden, E., & Michel, J. B. (2014). Uncharted: Big data as a lens on human culture. Penguin.
Allen, P. M., & Sanglier, M. (1979). A dynamic model of growth in a central place system. Geographical Analysis, 11(3), 256-272.
Andreu, L., Aldás, J., Bigné, J. E., & Mattila, A. S. (2010). An analysis of e-business adoption and its impact on relational quality in travel agency–supplier relationships. Tourism Management, 31(6), 777-787.
Berné, C., García-González, M., García-Uceda, M. E., & Múgica, J. M. (2015). The effect of ICT on relationship enhancement and performance in tourism channels. Tourism Management, 48, 188-198.
Boyd, D., & Crawford, K. (2011, September). Six provocations for big data. In A decade in internet time: Symposium on the dynamics of the internet and society (Vol. 21). Oxford: Oxford Internet Institute.
Buhalis, D., & Amaranggana, A. (2013). Smart tourism destinations. In Information and communication technologies in tourism 2014 (pp. 553-564). Springer, Cham.
Buhalis, D., & Amaranggana, A. (2015). Smart tourism destinations enhancing tourism experience through personalisation of services. In Information and Communication Technologies in Tourism 2015 (pp. 377-389). Springer, Cham.
Buhalis, D., & Law, R. (2008). Progress in information technology and tourism management: 20 years on and 10 years after the Internet—The state of eTourism research. Tourism management, 29(4), 609-623.
Chun, R. (2016, September, 22). Can Big Data Make for Better Exhibitions?, Artsy, Retrived from https://www.artsy.net/article/artsy-editorial-can-big-data-make-for-better-exhibitions
Corniani, M. (2002). Demand Bubble Management. SYMPHONYA, 1, 87-98.
Crouch, G. I., & Ritchie, J. B. (1999). Tourism, competitiveness, and societal prosperity. Journal of business research, 44(3), 137-152.
De Mauro, A., Greco, M., & Grimaldi, M. (2015, February). What is big data? A consensual definition and a review of key research topics. In AIP conference proceedings (Vol. 1644, No. 1, pp. 97-104). AIP.
Della Corte, V., & Aria, M. (2014). Why strategic networks often fail: some empirical evidence from the area of Naples. Tourism Management, 45, 3-15.
Della Corte, V., & Aria, M. (2016). Coopetition and sustainable competitive advantage. The case of tourist destinations. Tourism Management, 54, 524-540.
Franch, M. (Ed.). (2002). Destination management: governare il turismo tra locale e globale. Torino: Giappichelli Editore.
Fyall, A., Callod, C., & Edwards, B. (2003). Relationship marketing: The challenge for destinations. Annals of tourism research, 30(3), 644-659.
Getz, D. (2008). Event tourism: Definition, evolution, and research. Tourism management, 29(3), 403-428.
Laney, D. (2001). 3D data management: Controlling data volume, velocity and variety. META Group Research Note, 6, 70.
Lazer, D., Pentland, A. S., Adamic, L., Aral, S., Barabasi, A. L., Brewer, D., & Jebara, T. (2009). Life in the network: the coming age of computational social science. Science (New York, NY), 323(5915), 721.
Li, L., & Buhalis, D. (2006). E-Commerce in China: The case of travel. International Journal of Information Management, 26(2), 153-166.
Manovich, L. (2012). How to compare one million images?. In Understanding digital humanities (pp. 249-278). London: Palgrave Macmillan UK.
Mariani, M. M., Buhalis, D., Longhi, C., & Vitouladiti, O. (2014). Managing change in tourism destinations: Key issues and current trends. Journal of Destination Marketing & Management, 2(4), 269-272.
McAfee, A., & Brynjolfsson, E. (2012). Big data: the management revolution. Harvard business review, 90(10), 60-68.
Nalebuff, B. J., Brandenburger, A., & Maulana, A. (1996). Co-opetition. London: HarperCollinsBusiness.
Neal, J. D., & Gursoy, D. (2008). A multifaceted analysis of tourism satisfaction. Journal of Travel Research, 47(1), 53-62.
Nuccio, M., & Bertacchini E. (2016). Big Data Analytics in the arts and cultural industries: implication for cultural economics, policy and management. Paper Presented at the XIX ACEI Conference on Cultural Economics, Valladolid, Spain.
Palmer, A., & McCole, P. (2000). The role of electronic commerce in creating virtual tourism destination marketing organisations. International Journal of Contemporary Hospitality Management, 12(3), 198-204.
Pike, S. (2005). Tourism destination branding complexity. Journal of Product & Brand Management, 14(4), 258-259.
Pine, B. J., & Gilmore, J. H. (1999). The experience economy: work is theatre & every business a stage. Brighton: Harvard Business Press.
Rossi, C. (2008). Il management delle imprese di viaggio e turismo. Strategie e marketing (Vol. 2). Milano: FrancoAngeli.
Sashi, C. M. (2012). Customer engagement, buyer-seller relationships, and social media. Management decision, 50(2), 253-272.
Seddighi, H. R., & Theocharous, A. L. (2002). A model of tourism destination choice: a theoretical and empirical analysis. Tourism management, 23(5), 475-487.
Solima, L., Della Peruta, M. R., & Maggioni, V. (2016). Managing adaptive orientation systems for museum visitors from an IoT perspective. Business Process Management Journal, 22(2), 285-304.
Solima, L., Izzo, F., Mustillo, M. & Sasso. P. (2016). “Smart Tourism Destination from Iot Perspective: Adaptive Orientation System”, in Anriotis, K. Andriotis, K., Proceedings Of The International Conference On Tourism (Icot 2016). Paper presented in the International Conference On Tourism (Icot 2016). New Challenges and Boundaries in Tourism: Policies, Innovations and Strategies (654-666).
Solima, L. (2016). Smart Museums. Sul prossimo avvento della Internet of Things e del dialogo tra gli oggetti nei luoghi della cultura. Sinergie Italian Journal of Management, 99, 263-283.
Wang, D., Li, X. R., & Li, Y. (2013). China’s “smart tourism destination” initiative: A taste of the service-dominant logic. Journal of Destination Marketing & Management, 2(2), 59-61.
Ward, J. S., & Barker, A. (2013). Undefined by data: a survey of big data definitions. arXiv preprint arXiv:1309.5821.
Warnaby, G., Alexander, A., & Medway, D. (1998). Town centre management in the UK: a review, synthesis and research agenda. The International Review of Retail, Distribution and Consumer Research, 8(1), 15-31.
Wood, S. A., Guerry, A. D., Silver, J. M., & Lacayo, M. (2013). Using social media to quantify nature-based tourism and recreation. Scientific reports, 3, srep02976.
Xiang, Z., & Gretzel, U. (2010). Role of social media in online travel information search. Tourism management, 31(2), 179-188.
Yoshimura, Y., Sobolevsky, S., Ratti, C., Girardin, F., Carrascal, J. P., Blat, J., & Sinatra, R. (2014). An analysis of visitors’ behavior in the Louvre Museum: A study using Bluetooth data. Environment and Planning B: Planning and Design, 41(6), 1113-1131.